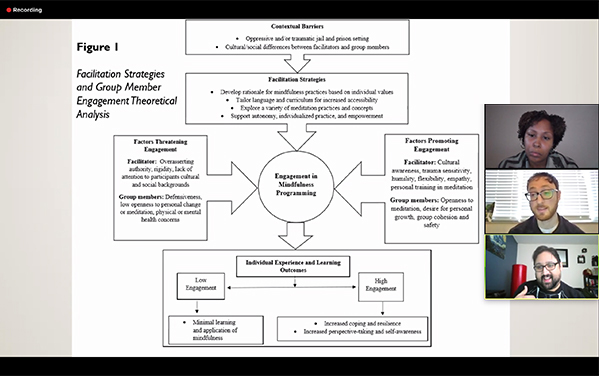
Online Ensemble Learning Phd Thesis, California Critical Thinking Test, Simi Valley Resume Com, 4 Lines English Writing Sheets/10() THE GUARANTEE Online Ensemble Learning Phd Thesis OF PRODUCTS’ UNIQUENESS. Our writers (experts, masters, bachelor, and doctorate) write all the papers from scratch and always follow the instructions of the client Online Ensemble Learning Phd Thesis/10() Online Ensemble Learning Phd Thesis a good essay yourself. You lack the motivation to research the topic. You lack the courage to submit the original text for review. You are eager Online Ensemble Learning Phd Thesis to learn from a professional to become seasoned in academic writing. You want an expert evaluation of your ideas and writings/10()
Online Ensemble Learning Phd Thesis
Machine Learning Modeling Research posted by Daniel Gutierrez, ODSC August 19, Daniel Gutierrez, online ensemble learning phd thesis, ODSC. As a data scientist, an integral part of my work in the field revolves around keeping current with research coming out of academia. I frequently scour arXiv. org for late-breaking papers that show trends and reveal fertile areas of research.
Other sources of valuable research developments are in the form of Ph. candidates are highly motivated to choose research topics that establish new and creative paths toward discovery in their field of study, online ensemble learning phd thesis.
Their dissertations are highly focused on a specific problem. If you can find a dissertation that aligns with your areas of interest, consuming the research is an excellent way to do a deep dive into the technology. After reviewing hundreds of recent theses from universities all over the country, I present 10 machine learning dissertations that I found compelling in terms of online ensemble learning phd thesis own areas of interest.
Each thesis may take a while to consume but will result in hours of satisfying summer reading. As we routinely encounter high-throughput data sets in complex biological and environmental research, developing novel models and methods for variable selection has received widespread attention.
This dissertation addresses a few key challenges in Bayesian modeling and variable selection for high-dimensional data with complex spatial structures. Big data vary in shape and call for different approaches. One type of big data is the tall data, i. This dissertation describes a general communication-efficient algorithm for distributed statistical learning on this type of big data.
The algorithm distributes the samples uniformly to multiple machines, and uses a common reference data to improve the performance of local estimates. The algorithm enables potentially much faster analysis, online ensemble learning phd thesis a small cost to statistical performance.
Another type of big data is the wide online ensemble learning phd thesis, i. It is also called high-dimensional data, to which many classical statistical methods are not applicable. This dissertation discusses a method of dimensionality reduction for high-dimensional classification.
The method partitions features into independent communities and splits the original classification problem into separate smaller ones. It enables parallel computing and produces more interpretable results.
The purpose of this machine learning dissertation is to address the following simple question:. How do we design efficient algorithms to solve optimization or machine learning problems where the decision variable or target label is a set of unknown cardinality?
Optimization and machine learning have proved remarkably successful in applications requiring the choice of single vectors. Some tasks, in particular many inverse problems, call for the design, or estimation, of sets of objects. When the size of these sets is a priori unknown, directly applying optimization or machine learning techniques designed for single vectors appears difficult.
The work in this dissertation shows that a very old idea for transforming sets into elements of a vector space namely, a space of measuresa common trick in theoretical analysis, generates effective practical algorithms.
Modern science and engineering often generate data sets with a large sample size and a comparably large dimension online ensemble learning phd thesis puts classic asymptotic theory into question in many ways. Therefore, the main focus of this dissertation is to develop a fundamental understanding of statistical procedures for estimation and hypothesis testing from a non-asymptotic point of view, where both the sample size and problem dimension grow hand in hand.
A range of different problems are explored in this thesis, including work on the geometry of hypothesis testing, adaptivity to local structure in estimation, effective methods for shape-constrained problems, and early stopping with boosting algorithms. The treatment of these different problems shares the common theme of emphasizing the underlying geometric structure. A random forest is a popular machine learning ensemble method that has proven successful in solving a wide range of classification problems.
While other successful classifiers, such as boosting algorithms or neural networks, admit natural interpretations as maximum likelihood, a suitable statistical interpretation is online ensemble learning phd thesis more elusive for a random forest. The first part of this dissertation demonstrates that a random forest is a fruitful framework in which to study AdaBoost and deep neural networks.
The work explores the concept and utility of interpolation, the ability of a classifier to perfectly fit its training data. The second part of this dissertation places a random forest on more sound statistical footing by framing it as kernel regression with the proximity kernel.
The work then analyzes the parameters that control the bandwidth of this kernel and discuss useful generalizations. A popular approach for relating correlated measurements of a non-Gaussian response variable to a set of predictors is to introduce latent random variables and fit a generalized linear mixed model.
The conventional strategy for specifying such a model leads to parameter estimates that must be interpreted conditional on the latent variables. In many cases, interest lies not in these conditional parameters, but rather in marginal parameters that summarize the average effect of the predictors across the entire population. Due to the structure of the generalized linear mixed model, the average effect across all individuals in a population is generally not the same as the effect for an average individual.
Further complicating matters, obtaining marginal summaries from a generalized linear mixed model often requires evaluation of an analytically intractable integral or use of an approximation. Another popular approach in this setting is to fit a marginal model using generalized estimating equations. This strategy is effective for estimating marginal parameters, but leaves one without a formal model for the data with which to assess quality of fit or make predictions for future observations.
Thus, there exists a need for a better approach. This dissertation defines a class of marginally interpretable generalized linear mixed models that leads to parameter estimates with a marginal interpretation while maintaining the desirable statistical properties of a conditionally specified model.
The distinguishing feature of these models is an additive adjustment that accounts for the curvature of the link function and thereby preserves a specific form for the marginal mean after integrating out the latent random variables, online ensemble learning phd thesis.
The objective of online ensemble learning phd thesis dissertation is to explore the use of machine learning algorithms in understanding and detecting hate speech, hate speakers and polarized groups in online social media. Beginning with a unique typology for detecting abusive language, online ensemble learning phd thesis, the work outlines the distinctions and similarities of different abusive language subtasks offensive language, hate speech, cyberbullying and trolling and how we might benefit from the progress made in each area, online ensemble learning phd thesis.
Serially correlated high dimensional data are prevalent in the big data era. In order to predict and learn the complex relationship among the multiple time series, high dimensional modeling has gained importance in various fields such as control theory, statistics, economics, finance, genetics and neuroscience. This dissertation studies a number of high dimensional statistical problems involving different classes of mixing processes, online ensemble learning phd thesis.
Random forest methodology is a nonparametric, online ensemble learning phd thesis, machine learning approach capable of strong performance in regression and classification problems involving complex data sets. In addition to making predictions, random forests can be used to assess the relative importance of feature variables. This dissertation explores three topics related to random forests: tree aggregation, variable importance, and robustness.
This dissertation solves two important problems in the modern analysis of big climate data. The second problem creates a way to supply the data for the technology developed in the first.
These two problems are computationally difficult, such as the representation of higher order spherical harmonics Y, which is critical for upscaling weather data to almost infinitely fine spatial resolution. Check out the Research Frontiers track at ODSC Europe this September or the ODSC West Research Frontiers track this October Daniel D.
As a technology journalist, he enjoys keeping a pulse on this fast-paced industry, online ensemble learning phd thesis. Daniel is also an educator having taught data science, machine learning and R classes at the university level. Be sure Modeling posted by ODSC Community Nov 22, In the Privacy-Enabling Online ensemble learning phd thesis PETs team at LiveRamp, we design and implement a wide range of AI seaborn Data Visualization posted by ODSC Team Nov 22, Data visualization is one of the most marketable and desirable data science skills out there.
php on line ODSC Conferences ODSC EAST ODSC WEST ODSC EUROPE ODSC APAC. Tools R Python Data Viz DataOps Platforms Workflow. ODSC Community Slack Channel ODSC Medium Publication Speaker Blogs Guest Contributors AI and Data Science News Research in academia Meetups. Dissertations for Machine Learning Modeling Research posted by Daniel Gutierrez, ODSC August 19, Daniel Gutierrez, ODSC. About author.
Daniel Gutierrez, ODSC Daniel D. LATEST POSTS View all. Privacy-Preserving Split Learning Modeling posted by ODSC Community Nov 22, In the Privacy-Enabling Technologies PETs team at LiveRamp, we design and implement a wide range of Teddy Petrou on Data Visualization Use Cases, Dash 2. POPULAR POSTS.
Machine Learning ODSC East Speaker Slides 64 East 48 Deep Learning 48 West 44 Accelerate AI 43 East 42 Conferences 41 Europe 39 Europe 37 West 34 R 34 West 33 NLP 31 AI 31 West 25 TensorFlow 24 Business 24 Reinforcement Learning 23 Python Related posts. Copyright © Open Data Science, online ensemble learning phd thesis. All rights reserved.
PhD Dissertation Defense - Faizan Shafique - Michigan State University l Zoom
, time: 33:37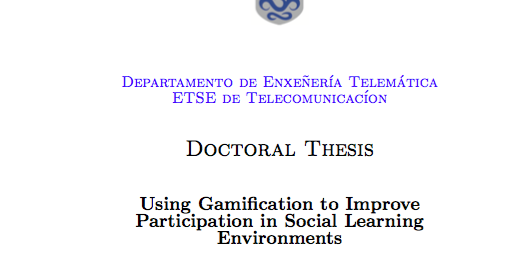
We will Online Ensemble Learning Phd Thesis not let you fail a class by missing the required deadline. Rely on the years of experience we have. Rely on the years of experience we have. There is no better way of solving Online Ensemble Learning Phd Thesis your writing problems than to visit our website/10() Online Ensemble Learning Phd Thesis, California Critical Thinking Test, Simi Valley Resume Com, 4 Lines English Writing Sheets/10() Online Ensemble Learning Phd Thesis, Top Essays Editing Site For Mba, Student Nurse Resume Help, Define Chronological Order In Essay. Buy Essay Online from the Best at a Reasonable Price. How to buy essay online from the best provider and ensure that the outcome meets the required quality standard for your college work/10()
No comments:
Post a Comment